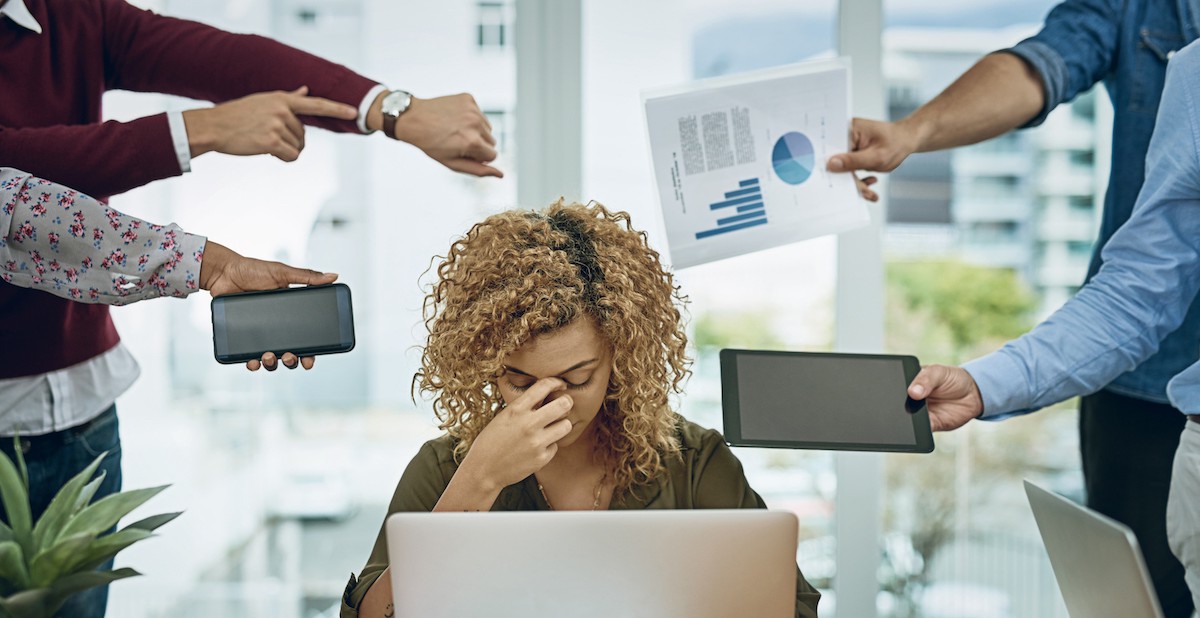
Cognitive Load Theory and Data Literacy
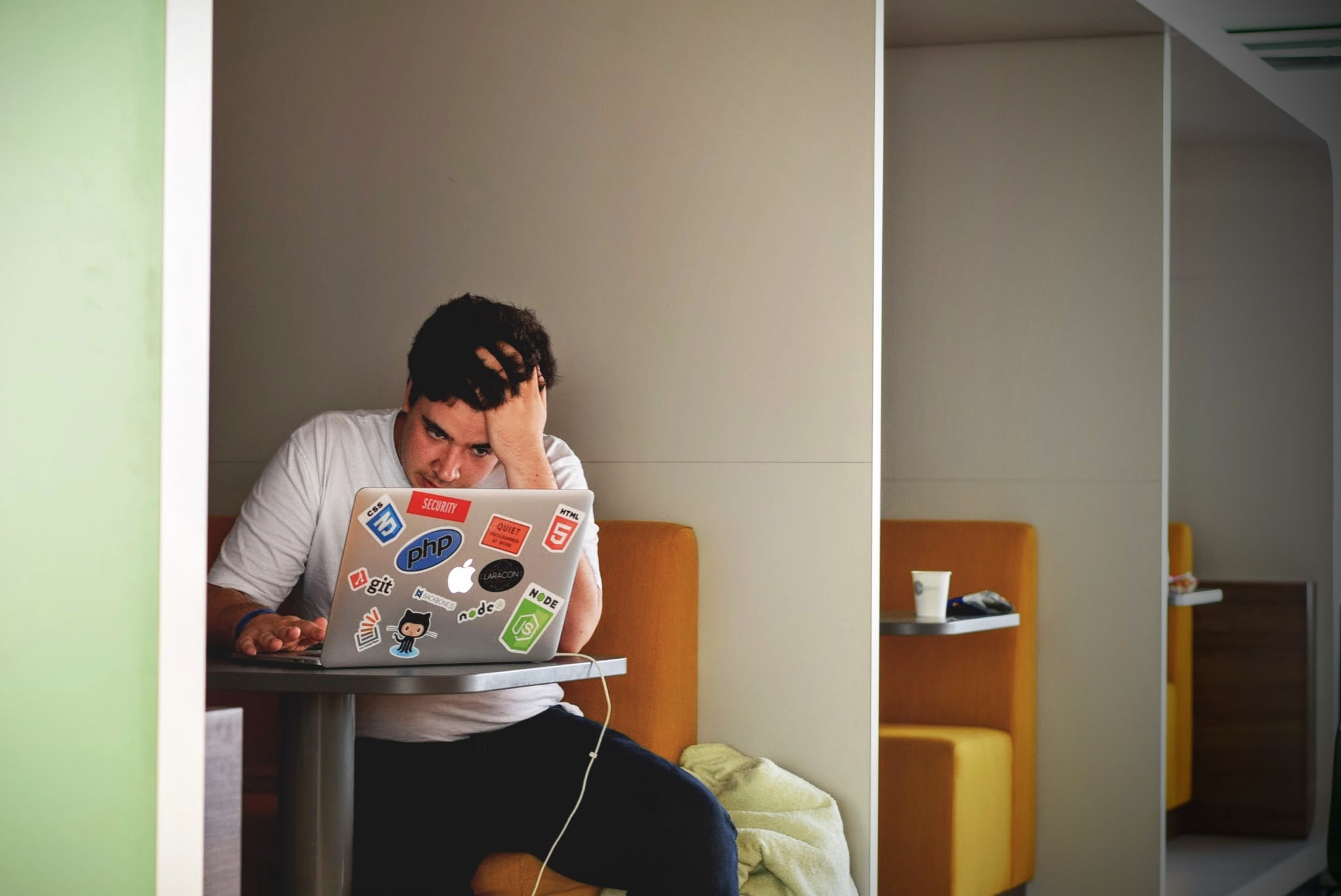
Remembering Data Insights: Strategy on Packaging Unforgettable Data Stories
Summary: Defining Exploratory and Explanatory Data Analysis and tips to implement for improved data story delivery.
Execution with data storytelling is – of course – going to dictate the success of any presentation.
Here’s the thing:
The final presentation is a sum of many parts. It’s not a matter of us taking a quick look at the numbers and then quickly trying to break it down to the data laymen of the world. Instead, we must rigorously immerse ourselves in the information and analyze it down to the last detail.
Furthermore, this deep dive must be performed, in part, through the lens of people outside of our discipline.
More specifically, our audience’s various industries have different uses and meanings for the data and information we’re presenting. Therefore, we mustn’t be one-dimensional with pre-analysis before explaining the stories being told by the numbers.
How we handle the raw data will dictate the way it’s presented. The two methods most commonly applied to this process are known as exploratory and explanatory data analysis.
What is Exploratory Data Analysis?
The definition of exploratory data analysis is almost precisely as it sounds. It encompasses all the ways that the information can be explored.
Exploratory analysis can begin with a hypothesis or question. We can also be examining the data to figure out what’s most enticing about it.
We must understand that raw data sets don’t tell us anything until they’ve been organized. This kind of curating or arranging can be done by utilizing an excel spreadsheet. Alternatively, if the available numbers allow for it, we can incorporate a more comprehensive visual model.

To delve deep into the ins-and-outs of data calls for our ability to point out trends, discrepancies, anomalies, and an array of other components. Doing so can only be done through the visualization and structuring the information we’re meant to interpret.
Here are a few considerations that should be applied to this process:
- Does a given visualization technique convey insights that others don’t?
- What differentiates the data point from the rest?
- What is the overall objective of the analysis, and how should it impact implementation into the story?
During this stage, we should see ourselves as “data detectives,” since the purpose is to suss out clues and evidence. Through this Sherlock Holmes-like, exploratory process, we learn how to pinpoint critical categories and build accuracy.
Neglecting this element of the job will only be to our detriment. Without performing this due diligence and putting in the “hard yards,” so to speak, it’s impossible to productively translate this information into tables, charts, or other visual forms.
The exploratory method is one of the most integral building blocks in creating viable algorithms and establishing deeper insights before implementing the data. It’s how we extract the proverbial data-diamonds from a rough that’s inundated with irrelevant information.
What is Explanatory Data Analysis?
Imagine we’ve completed the exploratory process, having calculated, modeled, or visualized all the statistics. Now, we have insights into the likelihood of specific events based on predetermined variables.

But what we don’t have is something that hones into the various contextual factors—namely, the “why” and “how.” This component is the explanatory data analysis, which helps with suggesting the soundest, most profitable actions. These insights are the entire point of a data story. Without the context or actionable plan, the whole presentation amounts to nothing but white noise.
Explanatory analyses are a frequent aspect of marketing—helping find out the reasons for various consumer behaviors.
The above insights can include why customers aren’t buying or why they’re likelier to buy specific items at given times during the year. For example, through the lessons learned from sound explanatory analysis, a marketer can decide with confidence whether to keep using the same social media campaign.
Conversely, marketers can keep digging into the numbers, find out about engagement and conversions, connect sales to unique demographics, and fine-tune the campaign to reach a bit further.
Finding a way to express data insights through the lens of “real life” is conducive to making more informed decisions, thus generating a higher return on investment.
Using These Methods in Practice
Hypothetically, let’s say we’re in charge of a shoe store, and have handed out customer satisfaction surveys. Our primary focus is any answer that falls below the “Very Satisfied” metric.
Here’s what the scale looks like, for reference:
- Very Dissatisfied – 1
- Dissatisfied – 2
- Neutral – 3
- Satisfied – 4
- Very Satisfied – 5
For this survey, we’re primarily concerned with customer service.
Under the lens of exploratory analysis, it might be wise to highlight everything short of “less than very satisfied” in red. We could arrange the data in descending order of this metric. The visual allows us to scan through the various issues and see the most relevant “less than very satisfied” score.
After extracting an insight to delve into, we can craft another visual representation to further highlight the point.
Or, in the interest of preserving the other data for context, we could alter the same visual to draw attention to the most crucial insights. An example of this could be changing the color of the information we want to stand out.
Whether we decide to isolate the most relevant data, or we merely choose to modify its appearance, attention is being taken away from the other numbers. Exploratory analysis ensures that we’re emphasizing the most valuable information that can give or audience the best possible outcome once we execute the explanatory phase.
Note that this example is highly simplified and used just to provide a baseline idea for how exploratory and explanatory data relate to one another.
Putting Data Stories to Work
As data storytellers, we’re only as useful as our insights are reliable, actionable, and profitable. This can’t happen if we don’t filter the information we’re meant to interpret through exploratory and explanatory data analysis (in that order).
These are the building blocks to success in any given data story—with each component being reliant on the other to generate the most engaging presentation. When combined together aptly and expertly, our audiences can then take the information and make their own form of magic.
Additional Resources
- Gestalt Principles and Storytelling with Data
- 6 Tips for Building Better Data Stories for Your Audience
- 17 Data Visualization Charting Types You Should Reference
Got Feedback or Wanna Talk?
Reach out using the form below:
[wpforms id=”185″ title=”false” description=”false”]